
Partition-based graph abstraction generates a topology-preserving map of single cells. With this, PAGA provides a graph abstraction method that is suitable for deriving interpretable abstractions of the noisy kNN-like graphs that are typically used to represent the manifolds arising in scRNA-seq data. Finally, we show how PAGA abstracts transition graphs, for instance, from RNA velocity and compare to previous trajectory-inference algorithms. Furthermore, we show that PAGA-initialized manifold learning algorithms converge faster, produce embeddings that are more faithful to the global topology of high-dimensional data, and introduce an entropy-based measure for quantifying such faithfulness. The data-driven formulation of PAGA allows to robustly reconstruct branching gene expression changes across different datasets and, for the first time, enabled reconstructing the lineage relations of a whole adult animal. Partition-based graph abstraction (PAGA) resolves these fundamental problems by generating graph-like maps of cells that preserve both continuous and disconnected structure in data at multiple resolutions.
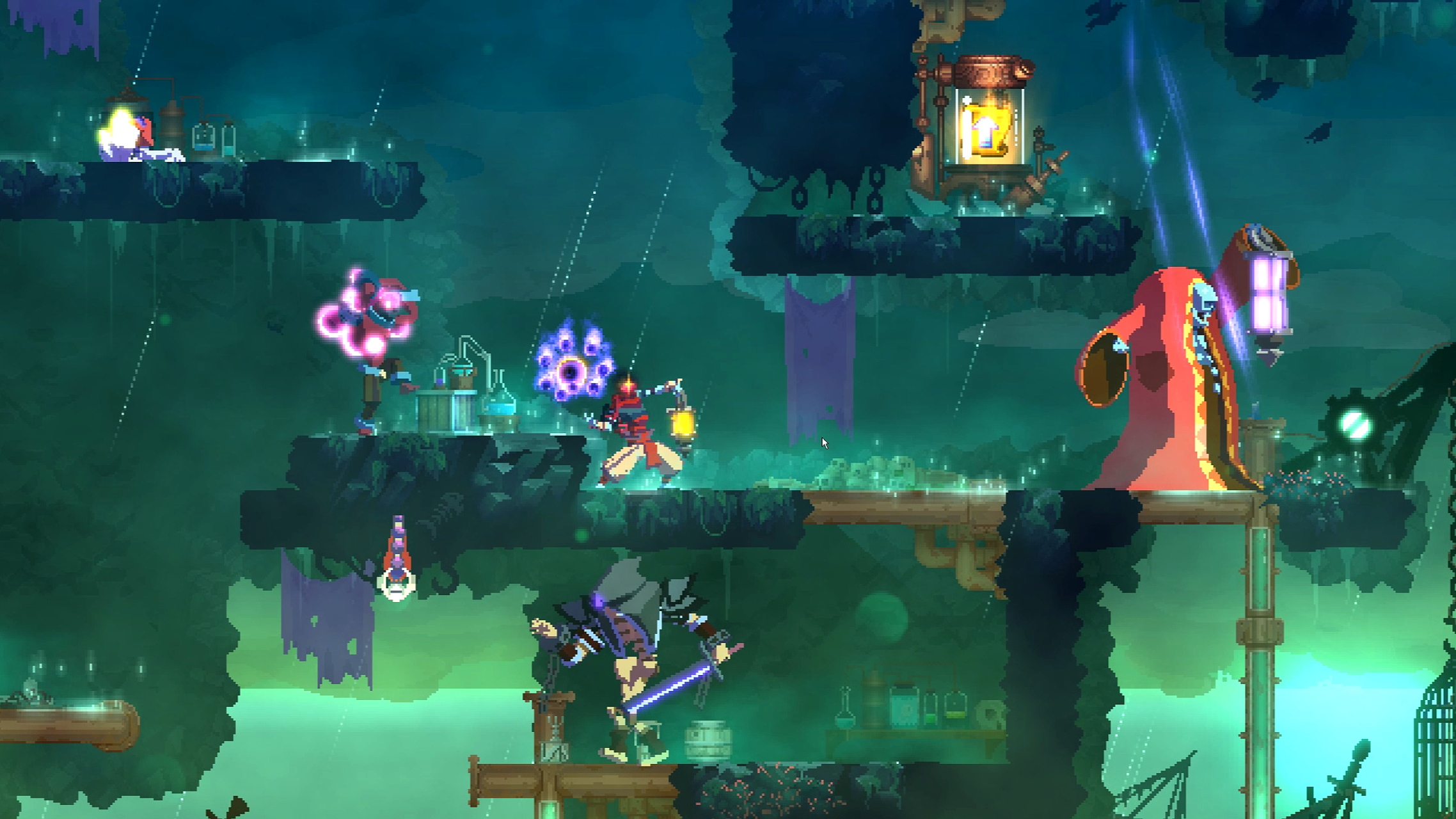
Efforts for addressing the resulting high non-robustness of tree-fitting to distances between clusters by sampling have only had limited success. However, such distance measures quantify biological similarity of cells only at a local scale and are fraught with problems when used for larger-scale objects like clusters. Moreover, they rely on feature-space based inter-cluster distances, like the euclidean distance of cluster means. This problem exists even in clustering-based algorithms for the inference of tree-like processes, which make the generally invalid assumption that clusters conform with a connected tree-like topology. As a consequence, experimental data do not conform with a connected manifold and the modeling of data as a continuous tree structure, which is the basis for existing algorithms, has little meaning. However, analyzing such data using pseudotemporal ordering faces the problem that biological processes are usually incompletely sampled. Here, we unify both viewpoints.Ī central example of dissecting heterogeneity in single-cell experiments concerns data that originate from complex cell differentiation processes. While the former approach is the basis for most analyses of single-cell data, the latter enables a better interpretation of continuous phenotypes and processes such as development, dose response, and disease progression. By contrast, inferring pseudotemporal orderings or trajectories of cells assumes that data lie on a connected manifold and labels cells with a continuous variable-the distance along the manifold. Clustering assumes that data is composed of biologically distinct groups such as discrete cell types or states and labels these with a discrete variable-the cluster index. Current computational approaches attempt to achieve this usually in one of two ways.
DEAD CELLS MAP CHARTS HOW TO
However, the algorithmic analysis of cellular heterogeneity and patterns across such landscapes still faces fundamental challenges, for instance, in how to explain cell-to-cell variation. The resulting datasets are often discussed using the term transcriptional landscape. Single-cell RNA-seq offers unparalleled opportunities for comprehensive molecular profiling of thousands of individual cells, with expected major impacts across a broad range of biomedical research.
